Lecturer | Prof. Dr. Jürgen Schmidhuber |
Module | Modul IN2064 |
Type | Lecture |
Language | English |
Semester | WS 2009/2010 |
ECTS | 6.0 |
SWS | 3V+2Ü |
Audience | Obligatory course for students of RCI Elective course for students of Informatics (Diplom 5+, Bachelor 5+, Master 1+) Elective course for students of Business Informatics (Bachelor 5+) |
Time & Location | Thu 10:00 - 12:00 MI HS 2 Fri 08:15 - 10:00 MI 00.08.038 |
Certificate | Final written exam: Sat, 06.02.2010, 10:30 - 12:30, MW1450 |
News

Tutorial
The tutorial consists of (mostly) weekly worksheets to be completed by the student, and discussion of the exercises. The worksheets will be posted here.Description
This lecture will take you on a journey through the exciting and highly active field of Machine Learning, which has applications in areas as diverse as web searches, robotics, data mining, environmental sciences, medical data analysis, and many more. The first part of the lecture loosely follows the textbook by Chris Bishop, referenced below, and uses a lot of his material. You are highly recommended to have a look into it for answers to fundamental questions, and for more in-depth information. Here is an overview of the topics covered (at least cursorily) by the lecture, where arrows indicate our flow of argument rather than historical derivation: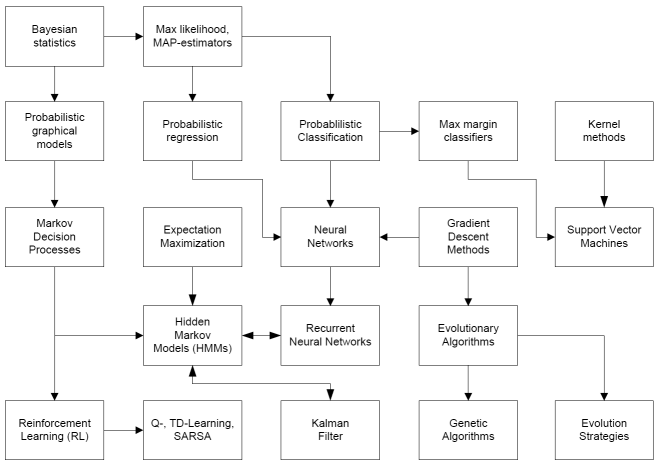
Material
Introduction (version of 23 Oct., 11:08 am: Minimal correction on page 28). Linear Regression and the additional information on validation sets. Linear Classification. Feed Forward Neural Networks. Introduction to Evolutionary Computing. Basic Algorithms Part 1, Part 2. Neuroevolution


Exercises
Exercises will be posted here. You need to solve at least 2/3 of the assignments to be allowed to the final exam. If you implement some of the algorithms you might want to have a look at a collection of data sets. Have a look at WEKA for implementations of machine learning algorithms. Stared exercises are optional. Assignment for 2009/10/22 (hand in on Thu, 29 Oct, before lecture). Assignment for 2009/10/29 (hand in on Thu, 5 Nov, before lecture). Have a look at the helpful notes by Sam Roweis. Assignment for 2009/11/05 (hand in on Thu, 12 Nov, before lecture). Assignment for 2009/11/12 (hand in on Thu, 19 Nov, before lecture). Assignment for 2009/11/19 (hand in on Thu, 10 Dec, before lecture). Assignment for 2009/12/17 (hand in on Thu, 7 Jan 2010, before lecture). Assignment for 2010/1/14 (hand in on Thu, 21 Jan 2010, before lecture). Assignment for 2010/1/21 (hand in on Thu, 28 Jan 2010, before lecture).Suggested Reading
[1] | David J. C. MacKay. Information theory, inference, and learning algorithms. Cambridge Univ. Press, 2008. |
[2] | Christopher M. Bishop. Pattern Recognition and Machine Learning. Springer, Berlin, New York, 2006. |
[3] | R.S. Sutton and A.G. Barto. Reinforcement Learning: An Introduction. MIT Press, 1998. |
[4] | Tom M. Mitchell. Machine learning. McGraw-Hill, Boston, Mass., 1997. |